The short-term rental vacation industry has evolved significantly over the past several years, moving from a niche mom-and-pop operation to a sophisticated, multi-billion dollar industry. It's also become much more important to depend on reliable technology in order to succeed in this business. Thankfully, there’ve been many advancements that are available to assist vacation rental managers and owners in their long lists of tasks.
This is specifically conspicuous in the realm of revenue management (RM) and pricing. While it’s a blessing that data is now so abundant, just having copious amounts isn’t an advantage if you don’t know what to do with it.
Fingertip access to this much data underscores the importance of utilizing algorithms, machine learning, and other automated technologies that matter to each vacation rental listing. Even the most experienced revenue managers simply cannot do these computations by themselves.
To benefit from advanced algorithms, you need to trust it.
And that starts with understanding what it’s actually doing, and how it’s working for you.
The Algorithm: It Starts With the Base Price
Beyond's dynamic pricing tool is powered by an algorithm that's really made up of several strains of complex logic, culminating in the correct daily price for each listing. The starting point of the “algo,” which we pioneered and affectionately refer to it as, is the Base Price.
This takes into account the “value” of the property, or the average price for your listing for the year. During periods of high demand, the algo will adjust upwards from the Base Price. It will do the same during low demand periods, lowering the price accordingly.
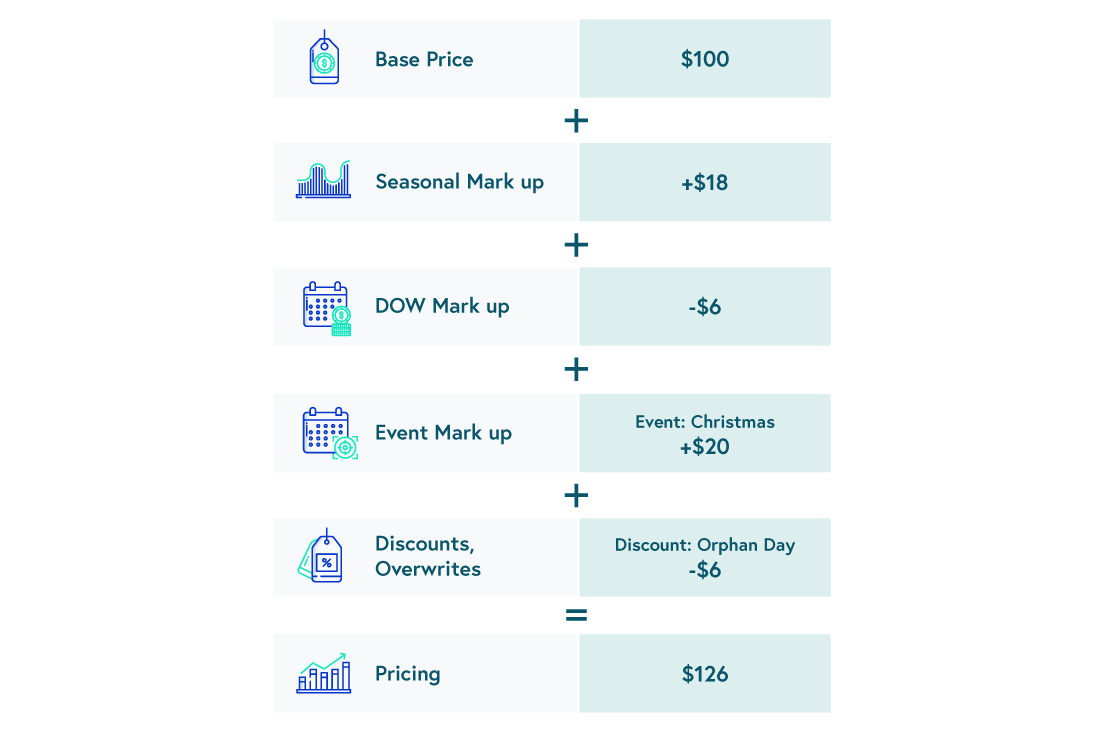
As pricing is all derived from the Base Price, getting it correct is very important. That’s why we even have secondary algorithms that help you set a base price based on the market and listing specific data.
As markets fluctuate—which this year has taught us in spades—it may be important to tweak the base price up or down slightly to keep the optimum price. Moving the base price is a simple way to effectively update your rates with one easy to use lever rather than trying to manipulate hundreds of rate rules.
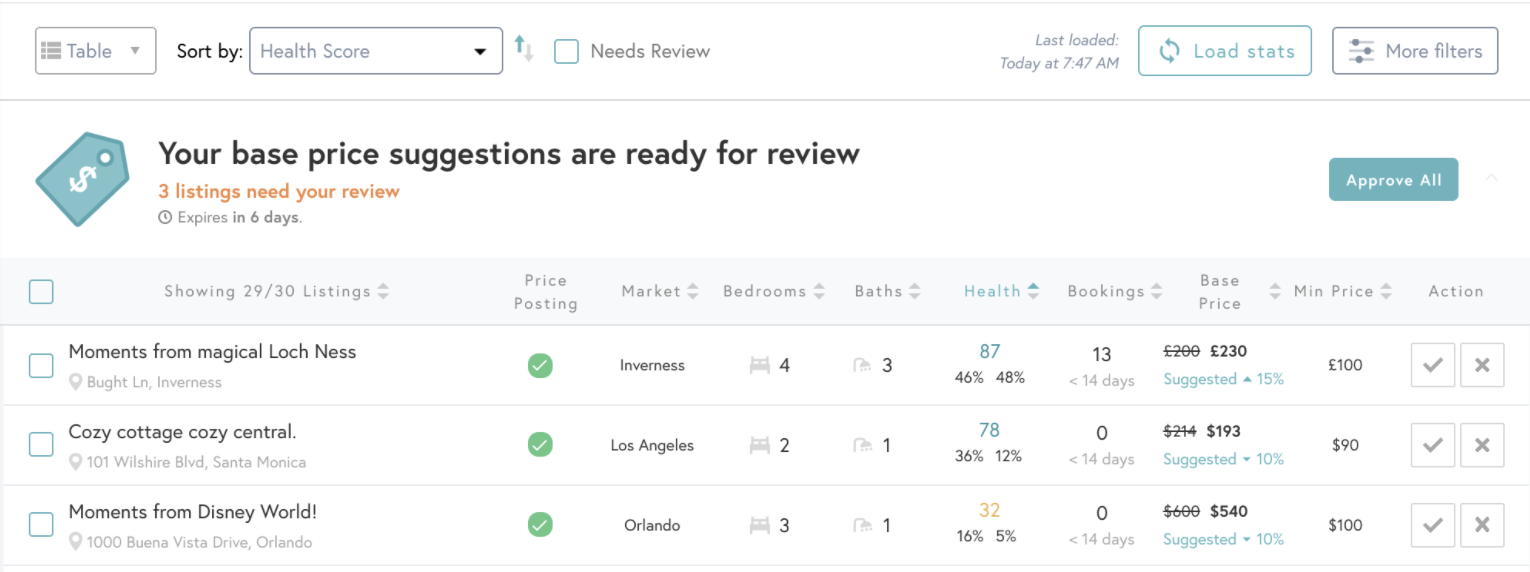
The Algo: Comp Sets
Once we’ve established the correct Base Price, it's now time to apply the algorithms to get daily prices. In order to do that, we need data—lots and lots of data! Our algorithms for daily pricing are powered from the continuous inflow of listing info, occupancy levels, and pricing data from Airbnb, VRBO and Booking.com.
Upon taking in this raw data, we repeatedly analyze and remove “bad” listings, ensuring the ones that do make it into the calculations are real, active, and accurate to use as acceptable data points.
Armed with data on millions of listings from multiple sources, we then formulate comp sets by taking “like units” in similar geographical areas and neighborhoods.
In other words, we look for between 100 and 500 similar listings and group them together.
While we have algorithms to automatically suggest comp set groups, especially as listings come and go from the OTAs, we also spend a painstaking amount of time making adjustments. If you’re a beach-front, ski on/off, or near a convention center, for example, you know that listings a few hundred feet away may have very different demand drivers and not actual comps.
The Algo: How We Get Daily Pricing Right
To get from the Base Price to daily prices that are shown to potential guests, we take the hyper-local data from our comp sets and extract trends around seasonality, day-of-the-week, and local events. Let's take a deeper look at what and why we do this:
Seasonality
To get the correct seasonality pricing, we use advanced decomposition logic to separate out seasonal trends and fluctuations that look back over the past 6+ years in most markets. Before you ask, we are obviously excluding data that breaks away from “normal” trends, like what we saw over the last eighteen months with the pandemic (i.e. just because April 2020 was awful doesn’t mean we will price April 2022 lower).
We factor in this seasonal trend for every hyper local area, which determines, based on demand, when and to what extent we increase prices for high, shoulder and low seasons. This is especially important as the distinction between these seasons isn’t black and white, but fluid. In most US summer markets, for example, the beginning and end of June are wildly different in their occupancy levels and rates—your prices need to ebb and flow along with that seasonality.
Same, Same, but Different
While this method works great for long standing historic norms, we understand that not every year is the same. Differences need to be not only expected but monitored and acted upon seamlessly. We also track “seasonal pacing” or certain times of the year that get higher or lower demand than previous years, of which Summer 2021 may be a prime example, given the pent-up demand we’ve seen.
In these cases, the algo tracks when and how much the demand differs and adjusts prices based on these pacing speed differences. Below is an example of pacing ahead in the Maine Coast.

Day of the Week
In addition to seasonality, the algorithm spends a lot of time making sure to get the proper day of the week (DoW) right, which can vary dramatically even in nearby areas. In a city, for example:
- The downtown area may have high DoW pricing on weekends near the bars and clubs,
- The financial district may actually have higher demand for Monday - Thursday,
- And the suburbs may be somewhere in between.
All of these factors need to be taken into consideration to get the right price.
Our algo runs subsequent decomposition to determine the relationship between the days of the week in order to set the right price. As anyone familiar with pricing will tell you, this relationship between days of the week is not constant and depends on the time of year. It must, therefore, be analyzed on a seasonal basis, not a static one.
The example below shows the DoW factor in South Lake Tahoe, where the deviation between the days of the week get significantly higher in winter with the arrival of weekend ski guests, and then shrinks in shoulder and summer seasons when guests stay longer on the lake.

Local Events
Events tend to be the hardest to price correctly and therefore deserve the most attention, which is why we have three algorithms simultaneously working to price all events appropriately.
The first algo uses the abundance of OTA data we have and continuously looks for day-over-day deviation in forward looking occupancy to spot increases in demand that aren’t caused by seasonality or DoW.
In the case of Panama City Beach below, for example, we can easily spot the increased occupancy from Labor Day, Fall Break, local events such as the Panama City Songwriters Festival and the Bloody May Music Festival, and of course Thanksgiving and Christmas.
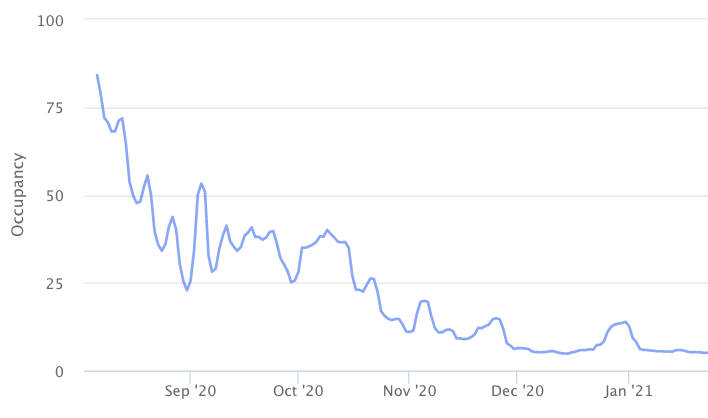
By continuously scanning future occupancy, the algo will increase the price as soon as there is an uptick, whether it’s from an expected national holiday or a one-off event like an eclipse or a concert.
The algo is always watching for future events so you don’t have to!
Using future demand to spot events is intuitive and makes sense, but for those with luxury listings or those who are in a market that book far out in advance, you may ask how do we price events very far out where demand hasn’t necessarily accumulated yet? Obviously you need your calendar to be open far in advance and can’t take low priced bookings.
This is where the algo gets even smarter and looks historically for the same known event to gauge the demand from previous years. It will then carry forward with a premium as a placeholder and floor until reservations catch up. Then, as we approach the event date, the historic demand blends to the future demand, so that in the rare cases where events are smaller than previous years, we won’t overprice.
Finally, as an additional algo, in the same way we monitor future occupancy, we’re also monitoring future prices of hotels (in addition to vacation rentals), and can use their pricing to detect any pricing anomalies, especially further away where reservation data is scarce. Having an algo that utilizes nearby hotel pricing to be aware of any developing trends in hotels that may affect the market allows us to be more confident in our pricing for every day of the year.
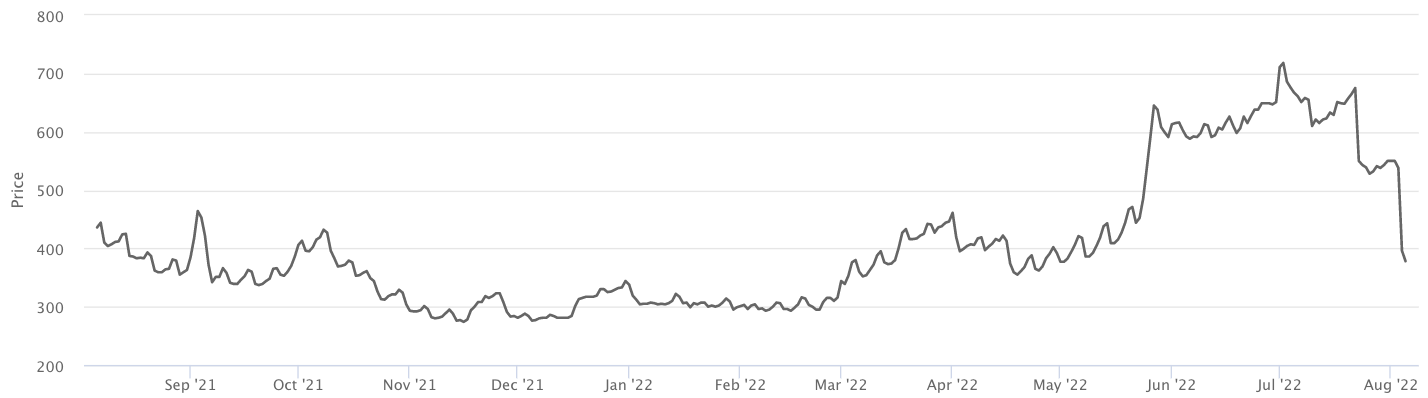
Conclusion
Hopefully this has given you a behind the scenes peek at how Beyond’s algorithm works for you. And as you’ve seen, there’s more than just one algo functioning! And we didn’t even cover the host of other supplemental algos like last-minute discounts, dynamic channel mark ups, gap filling and many others.
At the end of the day, the overlapping logics and algos we use are all working together to be your best partner in revenue management strategy—simplifying the overwhelming amount of data and making pricing decisions alongside you!
Ready to see how the algorithm can work for you? Try it here for free!